Research Areas
Solid-state Battery Materials
Understanding and designing novel materials for next-generation batteries through computational approaches and AI-driven discovery.
Strategic Solid-state Synthesis
Exploring reaction pathways and mechanisms in materials synthesis using advanced computational methods.
AI for Materials Discovery
Developing and applying machine learning models for accelerated materials discovery and property prediction.
Solid-state Battery Materials
Our research focuses on understanding and designing novel materials for next-generation batteries through computational approaches and AI-driven discovery. Key areas include:
- Investigation of ion transport mechanisms in solid electrolytes
- Design and optimization of new solid electrolyte materials
- Structure-property relationships in battery materials
Using advanced computational methods, including first-principles calculations and machine-learning-based molecular dynamics simulations, we aim to develop materials with enhanced ionic conductivity and stability for solid-state batteries.
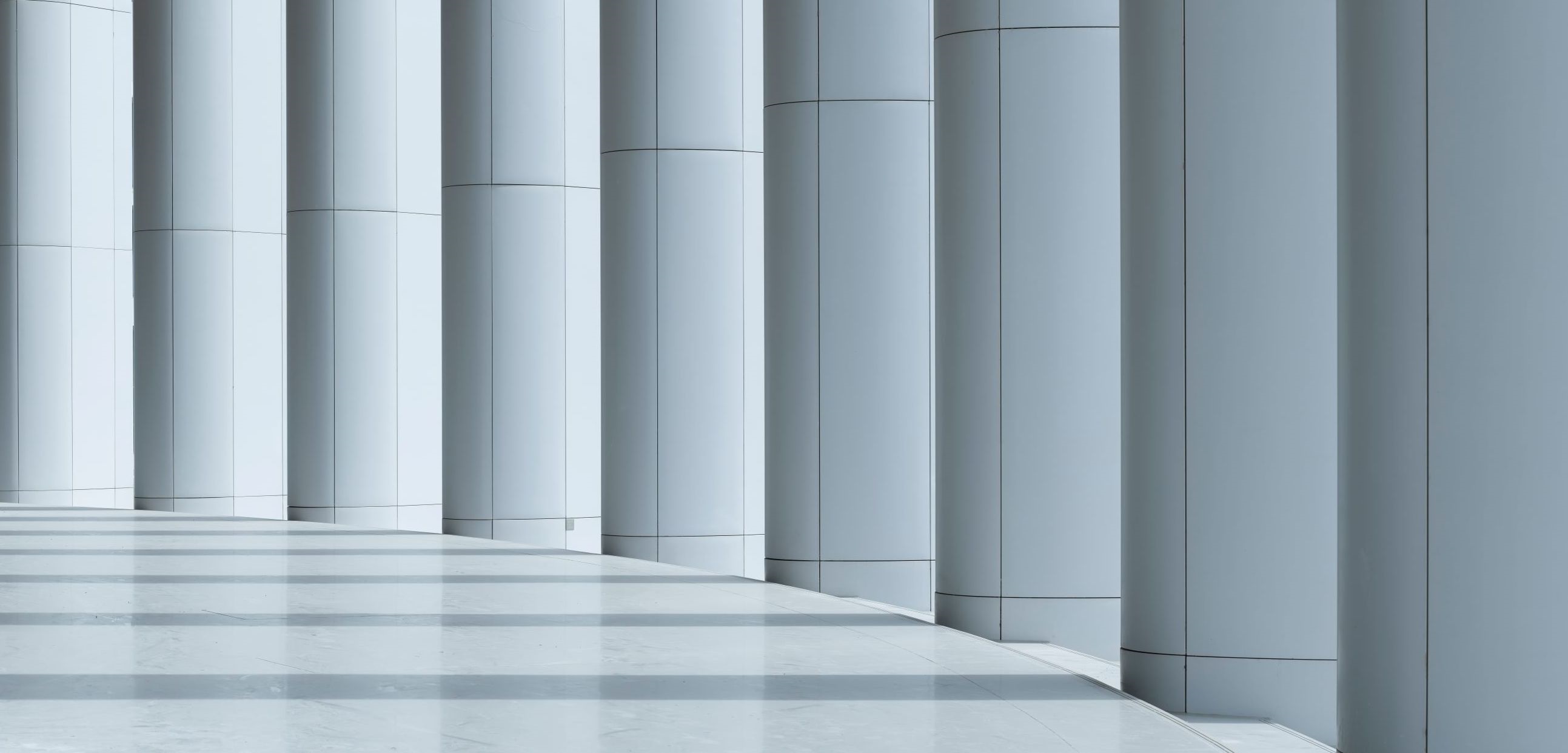
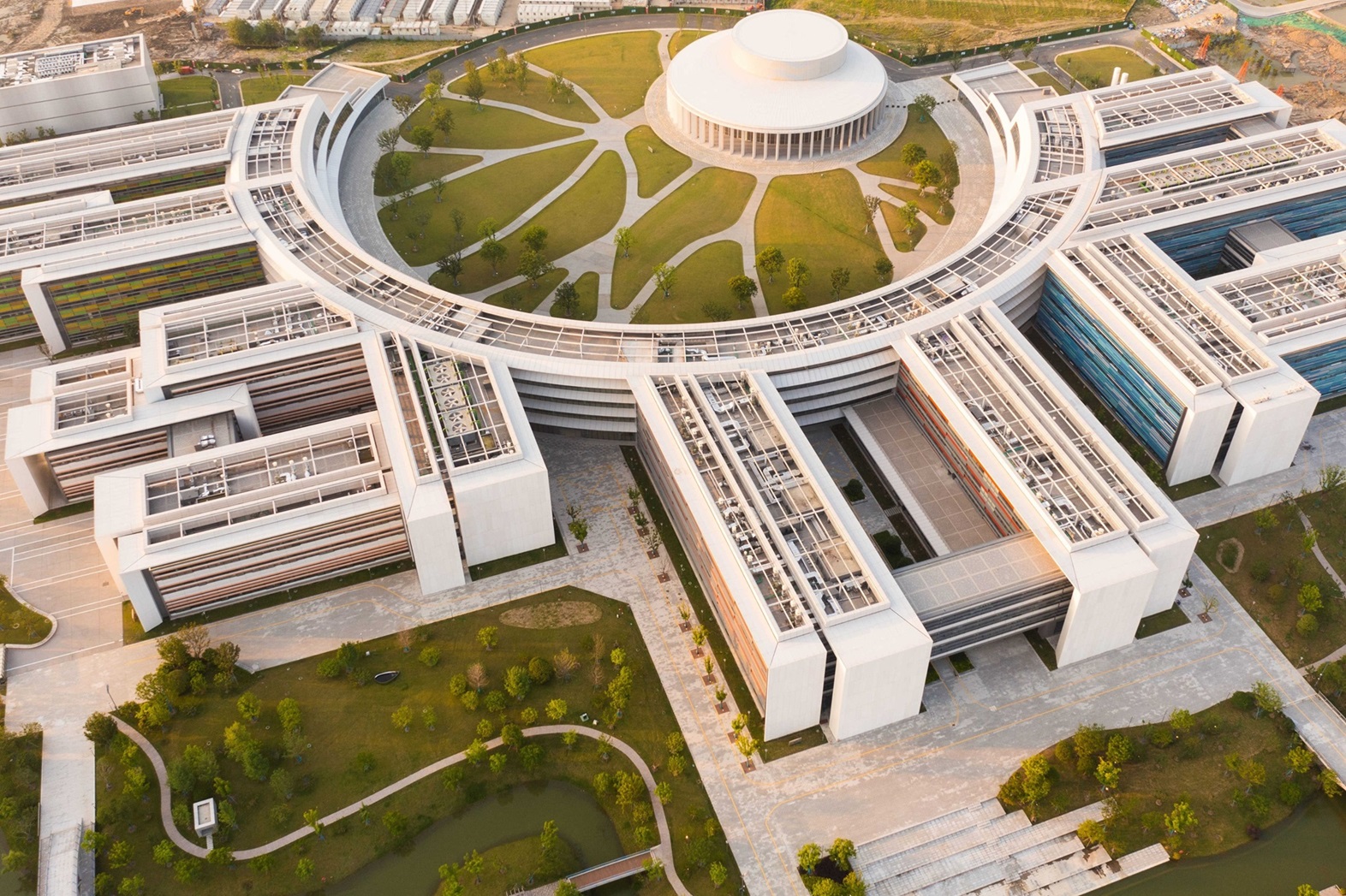
Strategic Solid-state Synthesis
We explore reaction pathways and mechanisms in materials synthesis using advanced computational methods. Our research includes:
- Prediction of synthesis conditions and reaction pathways
- Development of synthesis strategies for target materials
- High-throughput screening of synthesis conditions
By combining thermodynamic calculations with kinetic models, we aim to guide experimental synthesis and optimize processing conditions for novel materials.
AI for Materials Discovery
We develop and apply machine learning models for accelerated materials discovery and property prediction. Our focus areas include:
- Development of ML models for property prediction
- Automated materials screening and optimization
- Inverse design of materials with target properties
Through the combination of artificial intelligence and materials science principles, we aim to accelerate the discovery and development of new functional materials.
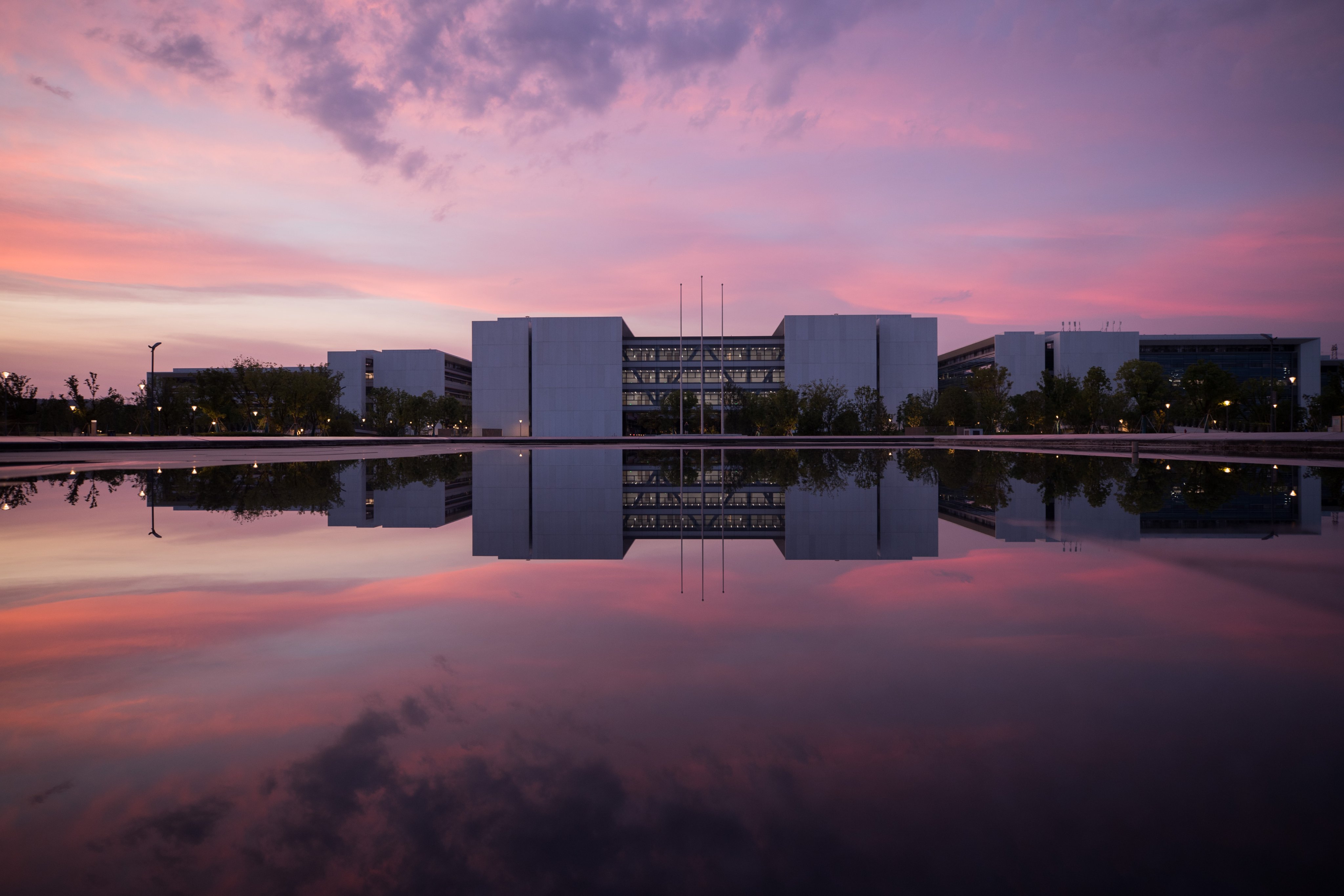